Pharmaceutical company improves OEE by 10% in 6 months by achieving maintenance excellence
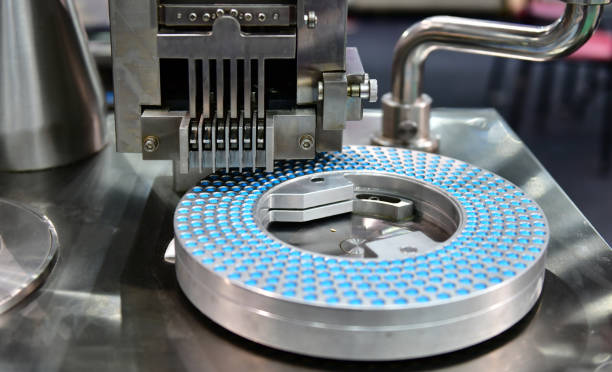
Discover how big data analytics can reduce your energy consumption.
It has become clear that the industrial sector is one of the largest consumers of energy in the world, accounting for over half of global energy consumption. The energy demand is expected to continue to grow as the world population increases and economies continue to develop.
In addition to this growing demand for energy and the global geopolitical situation, manufacturing companies face significant constraints, in which the fluctuation of the prices, the restrictive new regulations, and the market dynamics take the top 3 positions on the list.
The need to be adaptable as fast as possible to survive and maintain their competitive advantages became urgent, which can be done by:
In the following article, we explain how we are helping our partner optimize their energy consumption by leveraging data and advanced analytics. Furthermore, we will give you valuable insights on where and how to start your Digital Transformation Journey to reduce energy consumption.
Let’s make your data actionable!
To improve operations and competitiveness, and basically to survive in the future and stay ahead of the competition, companies need to focus on technological enablers, embracing SMART manufacturing process and having more control with the use of SMART sensors to generate data and perform advanced data analytics to get insights for a quicker decision making.
It’s important to emphasize that energy consumption is a major factor for high costs, especially in some assets-Intensive sectors.
Therefore, SMART manufacturing has become very competitive and valuable asset to upgrade the company’s business orientation (Business model, services.
By having greater control and visibility with a high level of granularity, the company can thrive during unstable periods by increasing productivity and quality, reducing energy consumption and costs.
Taking control over your consumption is a significant leverage to mitigate the restrictive regulation on CO2 emissions. Furthermore, SMART sensors can detect when industrial equipment are operating outside of its optimal range and adjust settings.
The project starts with product design, where AI can help you enhance your product design.
Data Analytics can help discover new materials and catalyzers (accelerators and enhancers) that can optimize production. It can also improve quality by having more intelligent Control Systems leading to less preparation.
Using, Advanced predictive maintenance of your machines will increase your efficiency by reducing the number of resources that are required and detecting inefficiencies such as heat losses. Furthermore, data analytics is the core of digital transformation initiatives.
Data analytics can, indeed, be considered an enabler of efficiency and optimization throughout the value chain:
For the client energy represents a very significant cost in their daily activities. To maintain their competitive advantage, they set goals and objectives which we will help them achieve.
For the client digital transformation in manufacturing starts with data from a broad range of sources and reduce manual entry and paper-based processes:
For the client, the value of this initiative is having the needed tools to answer questions in specific areas like:
Otofacto is one of the six founding partners of Collaboration Betters the World, a worldwide ecosystem of technology companies. Through technology, strategy and design, we help businesses grow. In order words, we advise, build, run technological solutions to support your company’s most important business challenges.
We use one methodology, two core teams, and are part of one ecosystem (CBTW). With this approach, we first assess your Industry 4.0 maturity. We listen to your needs. We align your people, processes, governance, and technologies to develop a tailor-made digital roadmap.
Our two core teams, Digital Core Team and Industrial Analytics Team, scope, develop, implement and maintain end-to-end digital solutions for your industrial environments.
As part of CBTW, we are leveraging our ecosystem of brands and partners to offer a complete range of transformative solutions.
In this project, the solution requested our client will include core features like:
In order to reach our objective, we will use a well-refined and tested process with 3 steps.
The main ingredient for our solution is “data” that describes the physical world’s situation which needs to be collected in its raw state and then converted, prepared, and contextualized to extract important information. Our client had installed sensors to gather this data.
We will analyze data from the shop floors and plants.
Turning data into actionable insights and identifying meaningful patterns represent one of the key challenges for many companies.
Throughout the process, continues and close cooperation between the engineers and data scientists exists in order to attain insights and patterns that are truly relevant.
After realizing the value from the insights, we implement the solution. We do this on a global scale, all plants and machines included! In order to do this on global scale, we deploy machines, configure, and adapt accordingly.
Undoubtedly, bringing interconnectivity between all machines and systems on a seemingly global scale, is one of the most challenging steps for many companies.
Furthermore, at this step, the engineers and the data scientists work closely together to bring the solution to the shop floor.
Conclusively, the client realizes the value at this step, as the desired results start pouring in and various objectives are achieved.
Overall, with advanced data analytics, companies can now transcend previously known constraints, such as the ability to control energy consumption, as discussed in the previous sections.
Otofacto is an expert in Industry 4.0 and has led many such initiatives of digital transformation strategies. Moreover, having the two core teams and being a part of the CBTW ecosystem enables Otofacto to tackle complex issues and find solutions efficiently.
The customer is already experiencing the added value from the project. In the future, more opportunities and profound results are likely to appear.