9 Common Challenges when implementing Operational Technology (OT) Systems
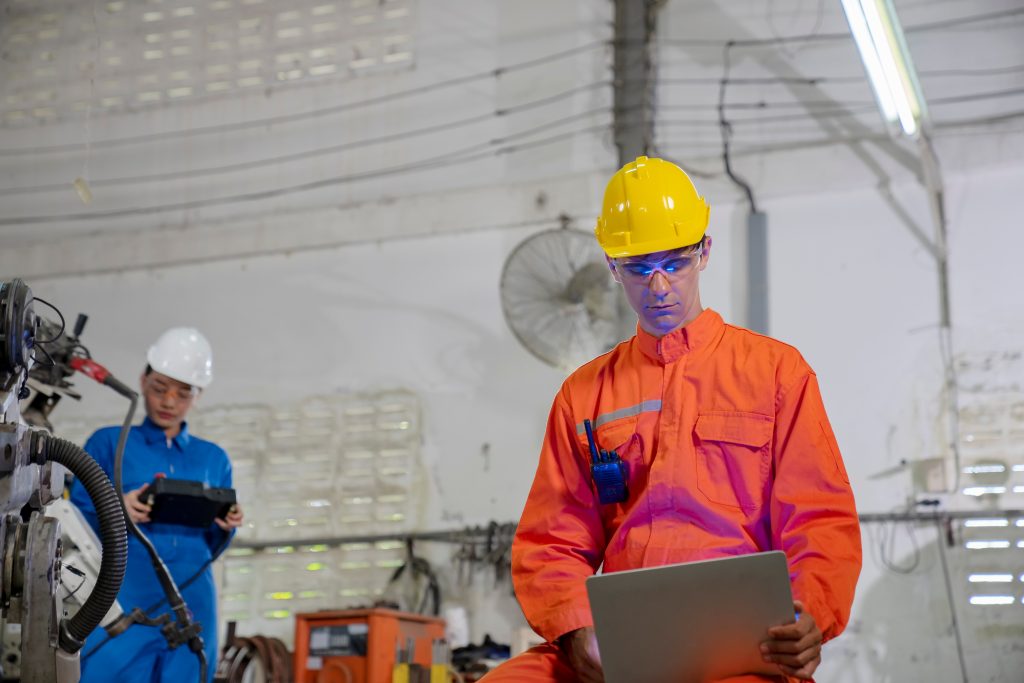
“Yeah hello, we’ve heard you are offering Industry 4.0 Analytics, can we buy this predictive maintenance solution? And if yes, when will you deliver it?”
Every. customer. ever.
In an exaggerated way, these first sentences of an introductory call by a new customer of our Analytics Consultancy represents the perception of predictive maintenance related projects spread all over the German market very well.
It’s always the same questions we are faced with when dealing with predictive maintenance:
After a few sessions, it most likely turns out that predictive maintenance, as the company trying to buy this mysterious predictive maintenance solution is calling it, should be replaced by a more general concept of machine learning methods brought to manufacturing businesses.
The practical truth is, that there is no one size fits all solution. Every project needs individual problem identification and solution, even if we are talking about the same use case for the 42th time.
Firstly, the real-world problems might differ from use case to use case.
For example, the underlying goals that should be addressed by a predictive maintenance solution:
Only a profound analysis of the real-world business and its underlying processes allows a well-fitting definition of the future target variables available in the data.
And this keyword brings us to the second point. We always have to match the data available with all its potential quality and availability issues to the real-world machine learning solution we want to build. And moreover, the Data Scientists have to translate and map the advanced analytics methods available for this future solution. This whole approach is the absolute necessary foundation for successful machine learning projects.
Predictive maintenance alone has many facets as we rarely predict the failure of a machine in the field in general, but, first put a lot of effort into the concretization of the very problem at hand.
In most cases, we predict not the complete failure of a machine but start with analyzing the failure of a few critical parts in order to find a promising starting point. In other cases, there is no historic failure information available and we cannot use predictive modelling. This is because it would be preferable, but we have to fall back on unsupervised learning methods for anomaly detection (usually in very high dimensional space with not so many extreme / unusual values we are hunting for). Nevertheless, it’s not only predictive maintenance with its challenges, manufacturing companies tackle the whole machine learning topic in most cases for the very first time. By doing that, they encounter very multilayered challenges regarding the establishment of a whole new professional service at the intersection of mathematics, computer science and process analysis.
To sum up, there obviously are common challenges all manufacturing companies are facing during their first contacts with machine learning. Although the buzzword “predictive maintenance” is on everyone’s lips, there still are no real best practices in the market. And besides predictive maintenance, there are plenty of (in most cases simpler!) machine learning use cases for manufacturing companies like predictive quality or production planning optimization.
In almost all cases, the professional relationships to our manufacturing customers started with a first request for a predictive maintenance solution and in all cases we started to interlace advanced analytics methods with the culture and DNA of the company in order to face the challenges during implementation of new data driven solutions.
Written by Caroline Kleist, Head of Data Science at Otofacto, Germany.